But in addition to identifying outliers we suggest some ways to better treat them. Generally Outliers affect statistical results while doing the EDA process we could say a quick example is the MEAN and MODE of a given set of data set which will be misleading that the data values would be higher than they really are.
10 How To Detect Outliers Data Science Beginners
When dealing with outliers discretion is required.
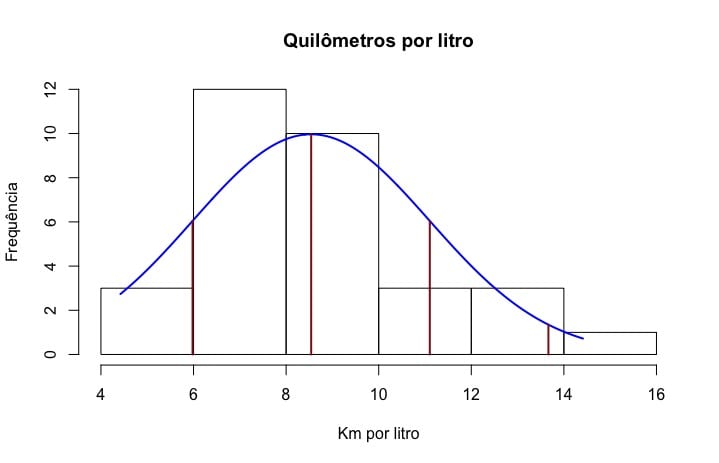
How to treat outliers in statistics. Given the problems they can cause you might think that its best to remove them from your data. Square root and log transformations both pull in high numbers. Unfortunately all analysts will confront outliers and be forced to make decisions about what to do with them.
Here 5 outliers is an acceptable amount of outliers however if we would have found 10 of outliers with 2 std then this could have meant that there are some natural extreme values such as. It also helps to have a clear understand of what your dataset describes in reality and what those outliers really represent how they came to be in existence. Outliers can now be detected by determining where the observation lies in reference to the inner and outer fences.
For the outer fences we start with the IQR and multiply it by 3. The same formula is also used in a boxplot. Using tukey formula to identify outlier.
For non-seasonal time series outliers are. One option is to try a transformation. This method has been dealt with in detail in the discussion about treating missing values.
Another way to handle true outliers is to cap them. 61 IQR Method. Drop the outlier records.
Using IQR we can find outlier. These data points which are way too far from zero will be treated as the outliers. Another way perhaps better in the long run is to export your post-test data and visualize it by various means.
In most of the cases a threshold of 3 or -3 is used ie if the Z-score value is greater than or less than 3 or -3 respectively that data point will be identified as outliers. Outlier on the lower side 1st Quartile 15 IQR. This technique uses the IQR scores calculated earlier to remove outliers.
You can compare the findings of the different methods and have confidence those data points can be treated as outliers when flagged by different methods independently. Exclude the discrepant observations from the data sample. This can make assumptions work better if the outlier is a dependent variable and can reduce the impact of a single point if the outlier is an independent variable.
Outliers are identified as points with residuals larger than U or smaller than L. Cap your outliers data. When the discrepant data is the result of an input error of the data then it needs to be removed from the sample.
Imputation with mean median mode. The CORRELATION COEFFICIENT is. The residuals are computed and the following bounds are computed.
Once the outliers are identified and you have decided to make amends as per the nature of the problem you may consider one of the following approaches. Outlier on the upper side 3rd Quartile 15 IQR. The first line of code below removes outliers based on the IQR range and stores the result in.
The tukey formula uses quantiles to produce upper and lower range values beyond which all values are considered as outliers. Based on different distributions and tools these can be categorised into two parts. 6 There are Two Methods for Outlier Treatment.
How to detect and treat outliers There are various methods to detect it. Then decide whether you want to remove change or keep outlier values. Really though there are lots of ways to deal with outliers.
Now how do we deal with outliers. Determine the effect of outliers on a case-by-case basis. U q 09 2 q 09 q 01 L q 01 2 q 09 q 01 where q 01 and q 09 are the 10th and 90th percentiles of the residuals respectively.
These two numbers are our outer fences. In statistics an outlier is a data point that significantly differs from the other data points in a sample. We then subtract this number from the first quartile and add it to the third quartile.
Here are four approaches. Outliers are unusual values in your dataset and they can distort statistical analyses and violate their assumptions. Often outliers in a data set can alert statisticians to experimental abnormalities or errors in the measurements taken which may cause them to omit the outliers from the data set.
Using the interquartile range IQR. The rule of thumb is that anything not in the range of Q1 - 15 IQR and Q3 15 IQR is an outlier and can be removed. In the case of Bill Gates or another true outlier sometimes its best to completely remove that record from your dataset to keep that person or event from skewing your analysis.
Outlier Detection With Boxplots In Descriptive Statistics A Box Plot By Vishal Agarwal Medium
Amazon Com Introduction To Regression Analysis Using R Easy Statistics Ebook Illukkumbura Anusha Kindle Store Regression Analysis Analysis Regression
7 1 6 What Are Outliers In The Data
Statistical Outliers Detection And Treatment Bista Solutions
5 Ways To Detect Outliers Anomalies That Every Data Scientist Should Know Python Code By Will Badr Towards Data Science
3 Methods To Deal With Outliers Data Science Machine Learning Method
What Are Outliers And How To Treat Them In Data Analytics Aquarela
8 Ways To Deal With Continuous Variables In Predictive Modeling Variables Continuity Data Science
0 comments:
Post a Comment